Jun 13, 2024
AI interface trends to pay attention to


In early 2010, responsive web design wasn’t a thing.
Then, almost overnight, designers were expected to become “mobile-native”.
A few weeks ago, Julius Tarng predicted history will repeat itself with AI.
So I’m inviting you to go on a journey with me to learn what it means to be an “AI-native” designer.
Let’s start by looking at some trends that are worth paying attention to 👇
Explaining the magic trick
AI is mostly a black box right now. Users hit a ✨button✨ and the AI spits out a magical answer.
Like any good magic trick you have absolutely no clue how it was done.
The more you rely on AI to perform complex actions, the more important it becomes to visualize what the heck the AI is doing behind the scenes.
That’s why I like this pattern from Elicit that breaks the AI’s output into a series of steps.
When users understand the processes involved, they’ll feel more confident in the result.

Giving more specific disclosure
“I think there is a lot of nuance around how much you want to label the AI output or how much human approval is needed or how you show people that AI might make mistakes sometimes.”
— Ryo Lu (designed Notion AI)
Right now you see a lot of generic disclaimers about AI’s propensity to make mistakes.
Typically it looks something like this subtext below the ChatGPT input 👀

But as AI becomes more integrated into your core product UX, blanket statements that push the liability to the AI aren’t going to cut it.
You’ll have to design more specific disclosure systems.
Here’s an example of how Maggie handles it in Elicit.
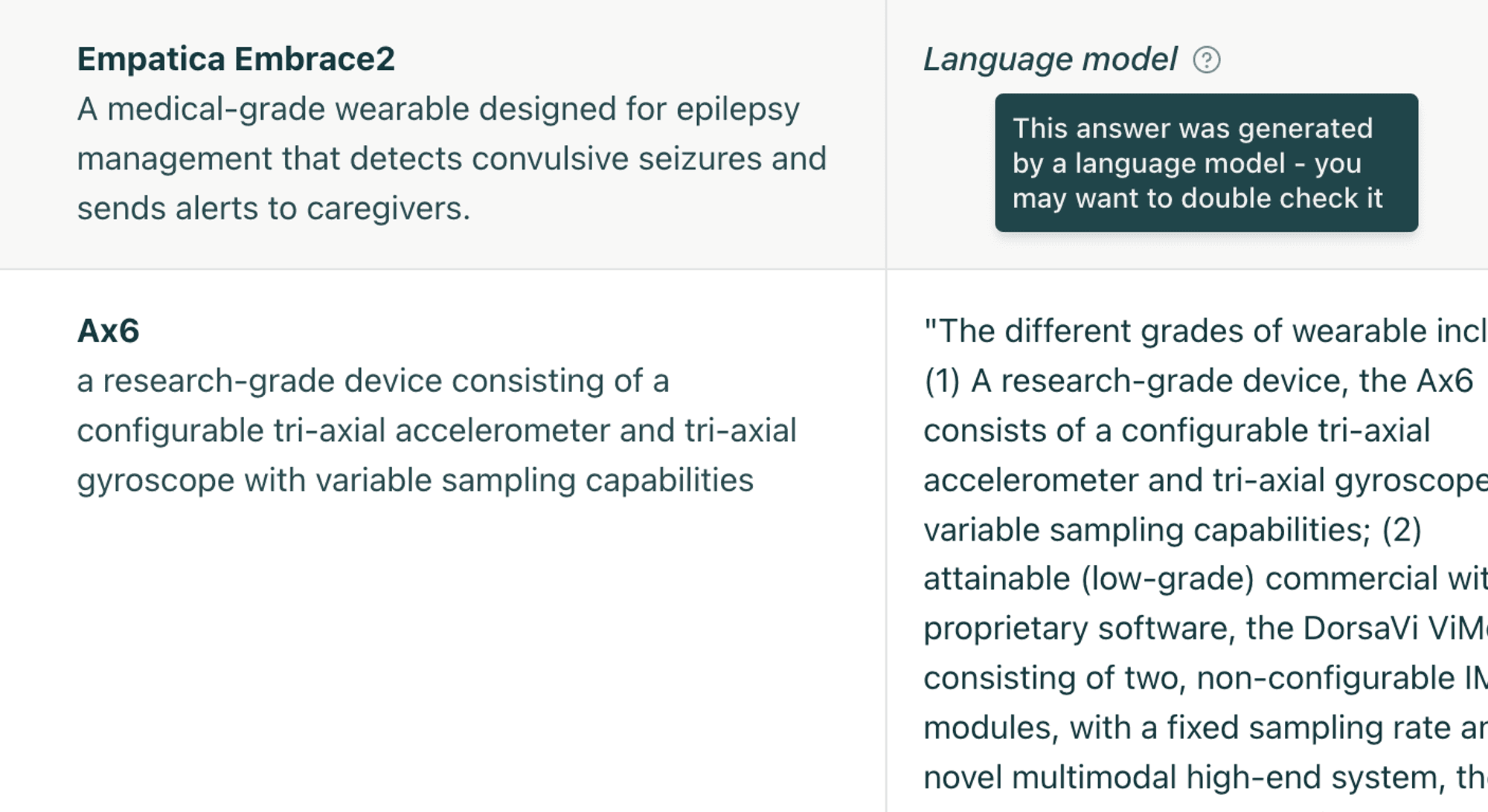
For context, Elicit makes it easy for scientists to analyze research papers and view the key results in custom tables. A single “don’t forget AI makes mistakes” at the table-level would be incredibly unhelpful.
Instead, she designed a disclosure system that lives at the result level.
If Elicit is confident that AI is referencing hard facts, it displays the citation with no disclaimer. But if the language model generates a response, Maggie discloses it in this tooltip.
Granular confidence scoring will be essential in AI-native interfaces.
Designing pointed UI patterns
As designers, it’s our responsibility to outline goal-oriented flows based on what we know about our users. The recent surge of chat-based UI doesn’t change that.
I’ll suggest it’s actually a failure of design to slap a chat UI on and say “here you go ask whatever you want!”
“Expecting users to primarily interact with software in natural language is lazy. It puts all the burden on the user to articulate good questions… to make sense of the response, and then to repeat that many times”
— Austin Henley
I’m much more excited about pointed applications for AI where the interaction is optimized for a focused purpose.
Not only is this easier for the user to understand, but constraining the types of prompts helps train the AI to deliver high quality responses.
One example I’ve seen in the wild recently is AI filtering from Dub.
I’m still typing in natural language, but the entry point is familiar and the interaction exists within the context of a specific user goal.

I also like some of the new features that Loom has been testing to streamline the process of creating written summaries.
They do a nice job of anticipating my needs and providing tailored affordances to help me achieve my goals (ex: writing a PR description).
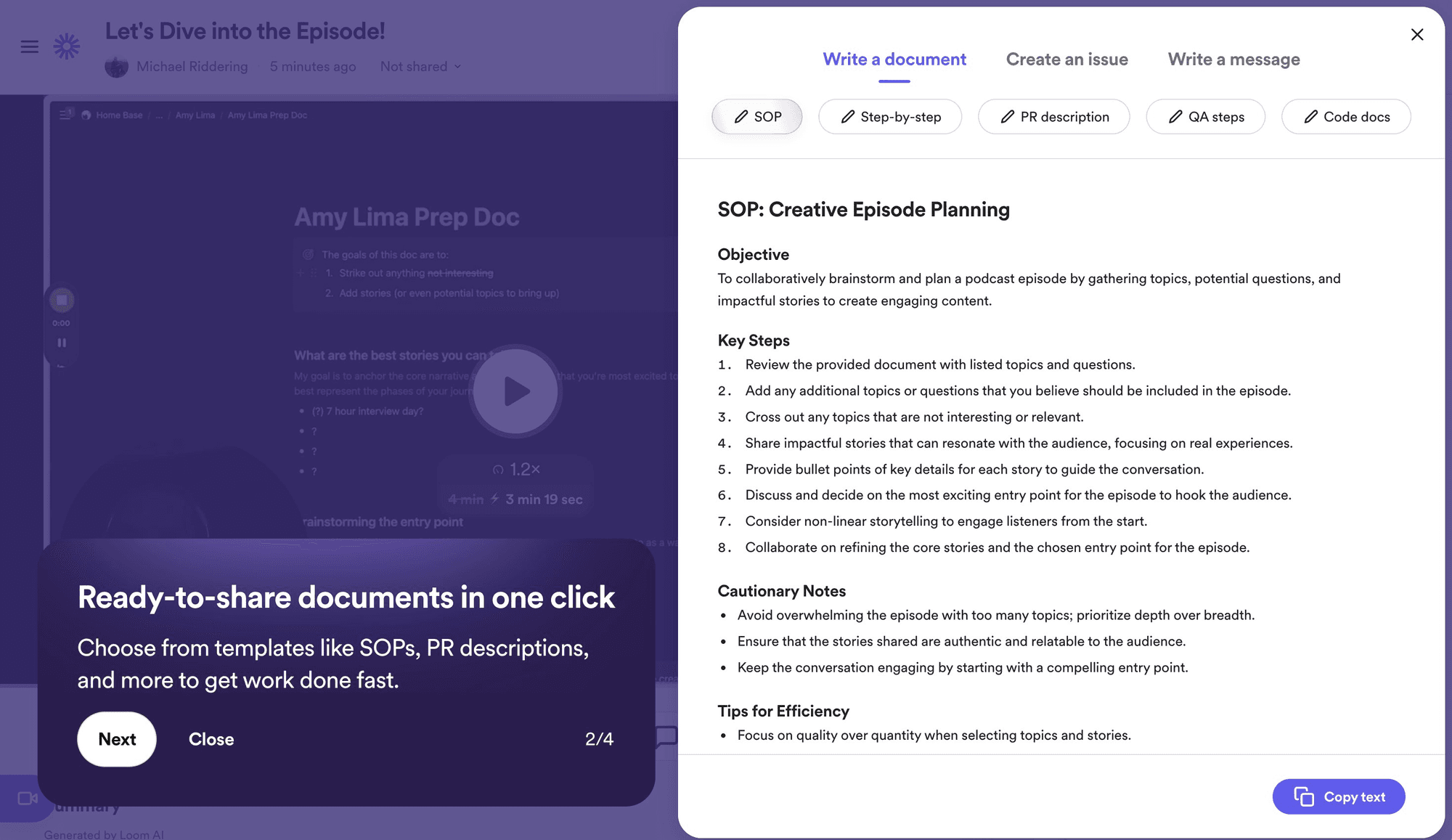
Becoming an AI-native designer
Maggie Appleton is the perfect person to show us what it's like designing for AI-native products.
As the first designer at Elicit (an AI assistant for research papers), she tackles all sorts of unique challenges that come with helping users interface with LLMs.
Plus her digital garden is one of my favorite places to be inspired.
So in this episode we go deep into:
How Maggie’s grown as a frontend developer
Strategies for improving your technical literacy
How writing online has impacted Maggie’s career
The AI-native tools that Maggie is drawing inspiration from
How Maggie’s newfound understanding of LLMs is shaping the way she designs
Why Maggie is more interested in the cognitive applications of AI rather than generative AI
Listen on YouTube, Spotify, Apple, or wherever you get your podcasts 👇
In early 2010, responsive web design wasn’t a thing.
Then, almost overnight, designers were expected to become “mobile-native”.
A few weeks ago, Julius Tarng predicted history will repeat itself with AI.
So I’m inviting you to go on a journey with me to learn what it means to be an “AI-native” designer.
Let’s start by looking at some trends that are worth paying attention to 👇
Explaining the magic trick
AI is mostly a black box right now. Users hit a ✨button✨ and the AI spits out a magical answer.
Like any good magic trick you have absolutely no clue how it was done.
The more you rely on AI to perform complex actions, the more important it becomes to visualize what the heck the AI is doing behind the scenes.
That’s why I like this pattern from Elicit that breaks the AI’s output into a series of steps.
When users understand the processes involved, they’ll feel more confident in the result.

Giving more specific disclosure
“I think there is a lot of nuance around how much you want to label the AI output or how much human approval is needed or how you show people that AI might make mistakes sometimes.”
— Ryo Lu (designed Notion AI)
Right now you see a lot of generic disclaimers about AI’s propensity to make mistakes.
Typically it looks something like this subtext below the ChatGPT input 👀

But as AI becomes more integrated into your core product UX, blanket statements that push the liability to the AI aren’t going to cut it.
You’ll have to design more specific disclosure systems.
Here’s an example of how Maggie handles it in Elicit.
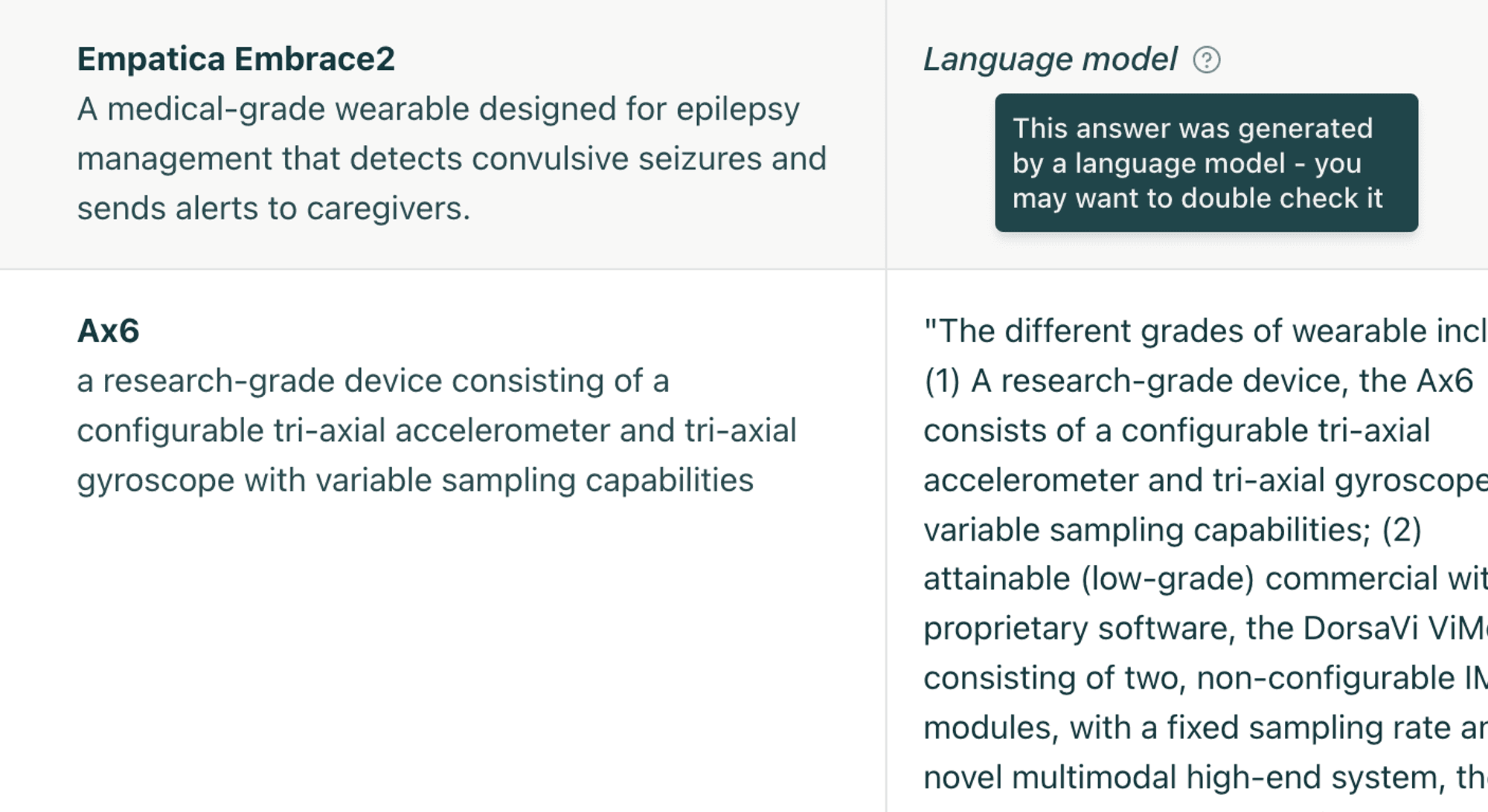
For context, Elicit makes it easy for scientists to analyze research papers and view the key results in custom tables. A single “don’t forget AI makes mistakes” at the table-level would be incredibly unhelpful.
Instead, she designed a disclosure system that lives at the result level.
If Elicit is confident that AI is referencing hard facts, it displays the citation with no disclaimer. But if the language model generates a response, Maggie discloses it in this tooltip.
Granular confidence scoring will be essential in AI-native interfaces.
Designing pointed UI patterns
As designers, it’s our responsibility to outline goal-oriented flows based on what we know about our users. The recent surge of chat-based UI doesn’t change that.
I’ll suggest it’s actually a failure of design to slap a chat UI on and say “here you go ask whatever you want!”
“Expecting users to primarily interact with software in natural language is lazy. It puts all the burden on the user to articulate good questions… to make sense of the response, and then to repeat that many times”
— Austin Henley
I’m much more excited about pointed applications for AI where the interaction is optimized for a focused purpose.
Not only is this easier for the user to understand, but constraining the types of prompts helps train the AI to deliver high quality responses.
One example I’ve seen in the wild recently is AI filtering from Dub.
I’m still typing in natural language, but the entry point is familiar and the interaction exists within the context of a specific user goal.

I also like some of the new features that Loom has been testing to streamline the process of creating written summaries.
They do a nice job of anticipating my needs and providing tailored affordances to help me achieve my goals (ex: writing a PR description).
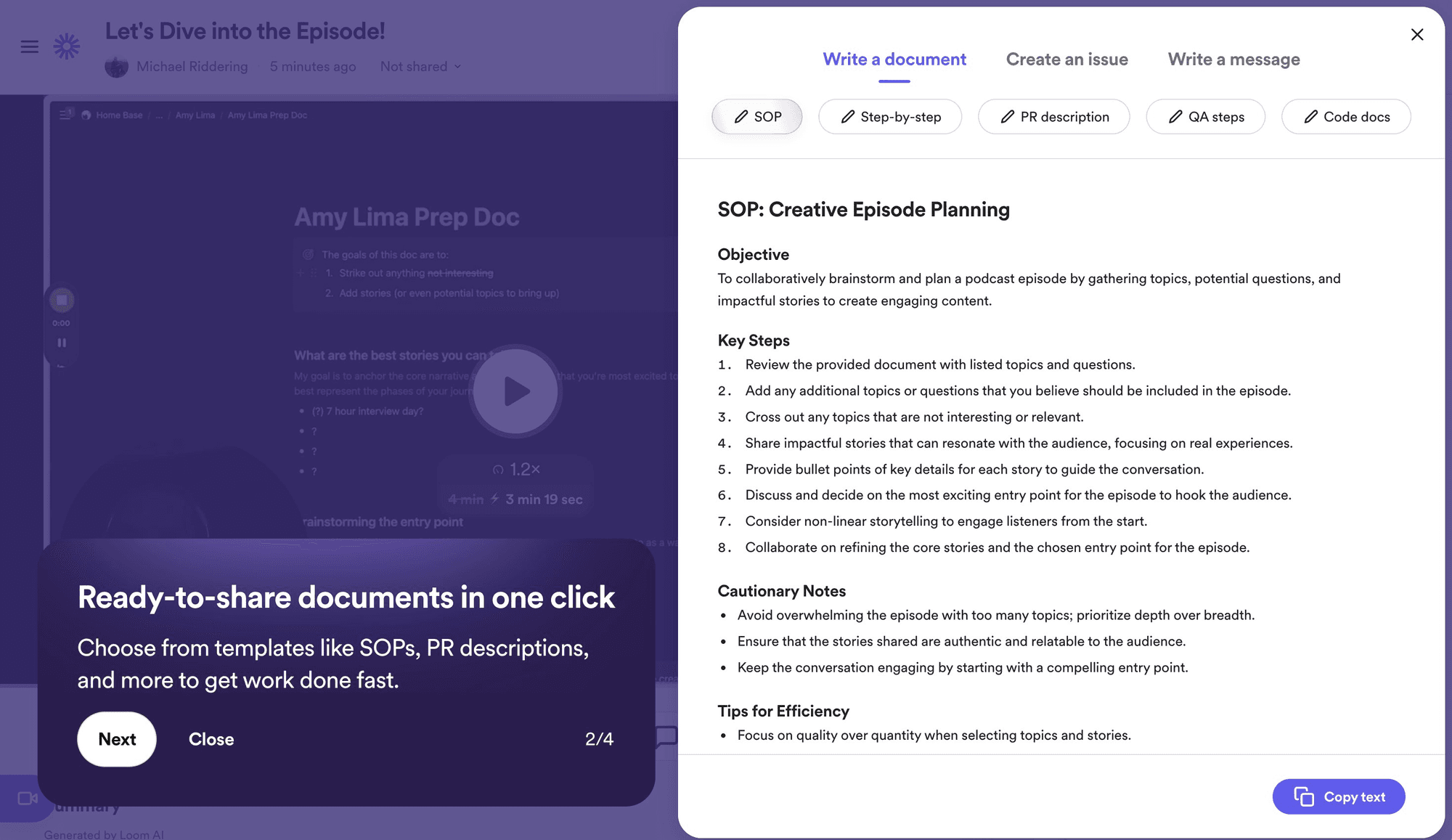
Becoming an AI-native designer
Maggie Appleton is the perfect person to show us what it's like designing for AI-native products.
As the first designer at Elicit (an AI assistant for research papers), she tackles all sorts of unique challenges that come with helping users interface with LLMs.
Plus her digital garden is one of my favorite places to be inspired.
So in this episode we go deep into:
How Maggie’s grown as a frontend developer
Strategies for improving your technical literacy
How writing online has impacted Maggie’s career
The AI-native tools that Maggie is drawing inspiration from
How Maggie’s newfound understanding of LLMs is shaping the way she designs
Why Maggie is more interested in the cognitive applications of AI rather than generative AI
Listen on YouTube, Spotify, Apple, or wherever you get your podcasts 👇
In early 2010, responsive web design wasn’t a thing.
Then, almost overnight, designers were expected to become “mobile-native”.
A few weeks ago, Julius Tarng predicted history will repeat itself with AI.
So I’m inviting you to go on a journey with me to learn what it means to be an “AI-native” designer.
Let’s start by looking at some trends that are worth paying attention to 👇
Explaining the magic trick
AI is mostly a black box right now. Users hit a ✨button✨ and the AI spits out a magical answer.
Like any good magic trick you have absolutely no clue how it was done.
The more you rely on AI to perform complex actions, the more important it becomes to visualize what the heck the AI is doing behind the scenes.
That’s why I like this pattern from Elicit that breaks the AI’s output into a series of steps.
When users understand the processes involved, they’ll feel more confident in the result.

Giving more specific disclosure
“I think there is a lot of nuance around how much you want to label the AI output or how much human approval is needed or how you show people that AI might make mistakes sometimes.”
— Ryo Lu (designed Notion AI)
Right now you see a lot of generic disclaimers about AI’s propensity to make mistakes.
Typically it looks something like this subtext below the ChatGPT input 👀

But as AI becomes more integrated into your core product UX, blanket statements that push the liability to the AI aren’t going to cut it.
You’ll have to design more specific disclosure systems.
Here’s an example of how Maggie handles it in Elicit.
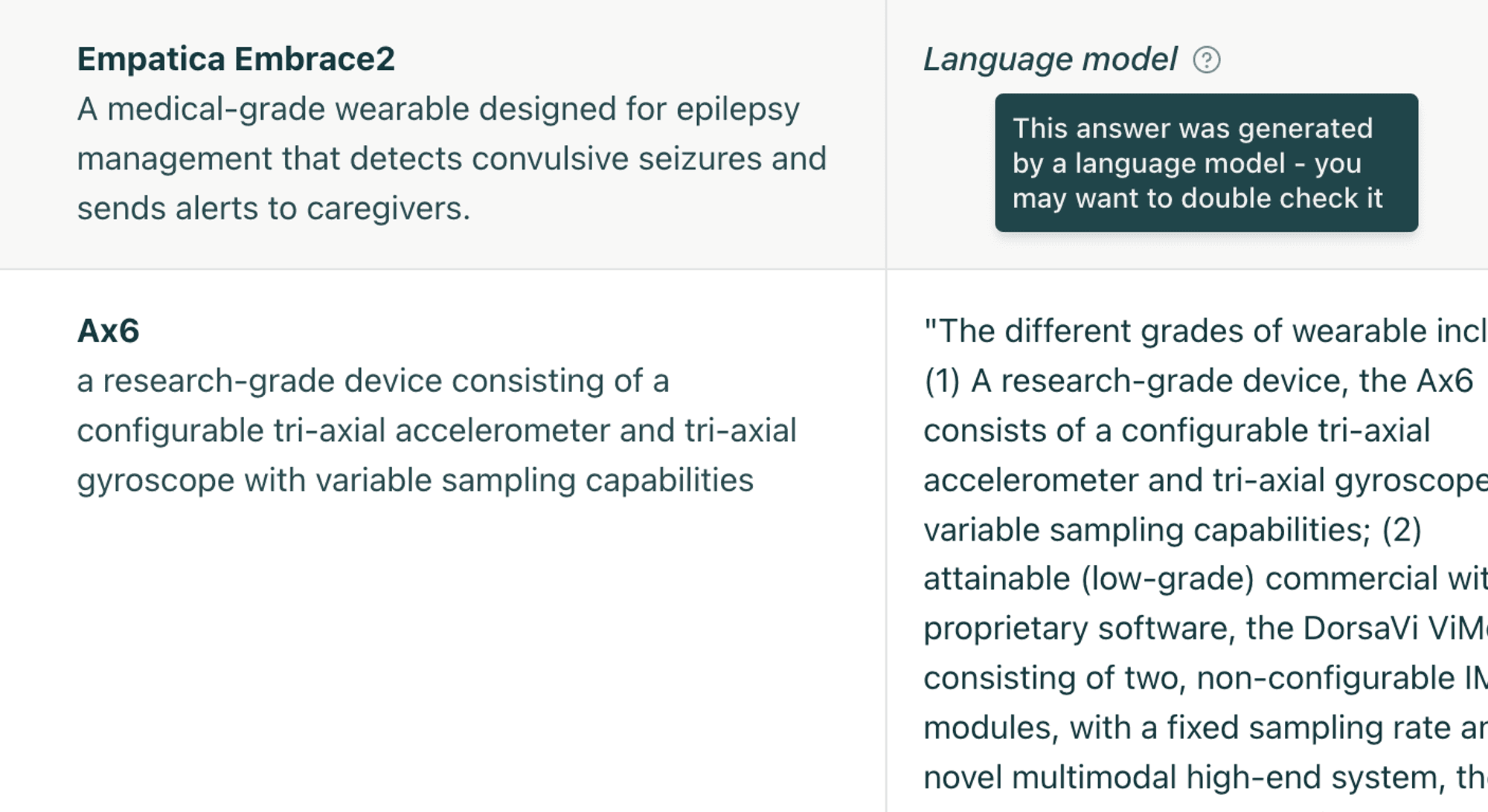
For context, Elicit makes it easy for scientists to analyze research papers and view the key results in custom tables. A single “don’t forget AI makes mistakes” at the table-level would be incredibly unhelpful.
Instead, she designed a disclosure system that lives at the result level.
If Elicit is confident that AI is referencing hard facts, it displays the citation with no disclaimer. But if the language model generates a response, Maggie discloses it in this tooltip.
Granular confidence scoring will be essential in AI-native interfaces.
Designing pointed UI patterns
As designers, it’s our responsibility to outline goal-oriented flows based on what we know about our users. The recent surge of chat-based UI doesn’t change that.
I’ll suggest it’s actually a failure of design to slap a chat UI on and say “here you go ask whatever you want!”
“Expecting users to primarily interact with software in natural language is lazy. It puts all the burden on the user to articulate good questions… to make sense of the response, and then to repeat that many times”
— Austin Henley
I’m much more excited about pointed applications for AI where the interaction is optimized for a focused purpose.
Not only is this easier for the user to understand, but constraining the types of prompts helps train the AI to deliver high quality responses.
One example I’ve seen in the wild recently is AI filtering from Dub.
I’m still typing in natural language, but the entry point is familiar and the interaction exists within the context of a specific user goal.

I also like some of the new features that Loom has been testing to streamline the process of creating written summaries.
They do a nice job of anticipating my needs and providing tailored affordances to help me achieve my goals (ex: writing a PR description).
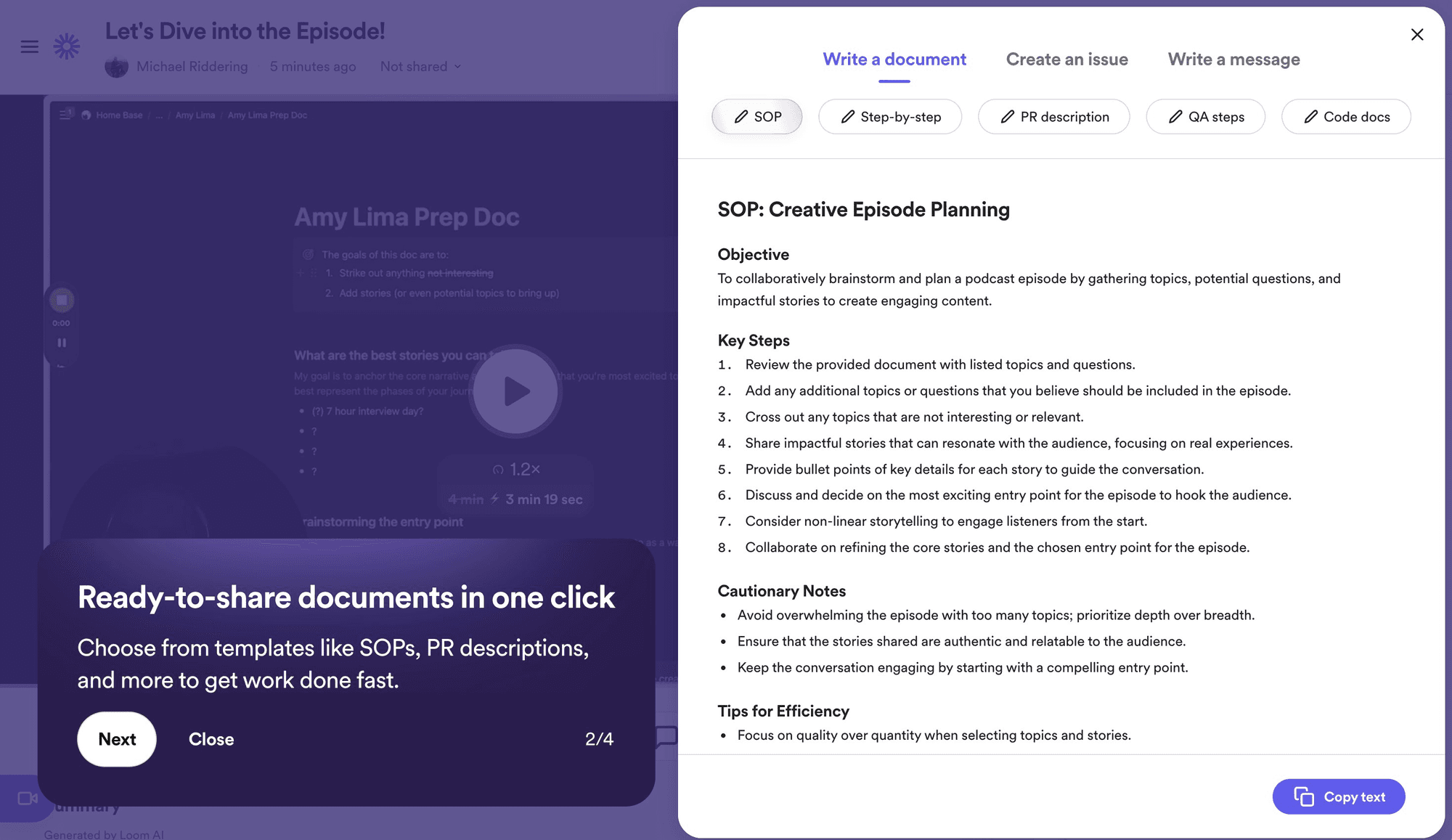
Becoming an AI-native designer
Maggie Appleton is the perfect person to show us what it's like designing for AI-native products.
As the first designer at Elicit (an AI assistant for research papers), she tackles all sorts of unique challenges that come with helping users interface with LLMs.
Plus her digital garden is one of my favorite places to be inspired.
So in this episode we go deep into:
How Maggie’s grown as a frontend developer
Strategies for improving your technical literacy
How writing online has impacted Maggie’s career
The AI-native tools that Maggie is drawing inspiration from
How Maggie’s newfound understanding of LLMs is shaping the way she designs
Why Maggie is more interested in the cognitive applications of AI rather than generative AI
Listen on YouTube, Spotify, Apple, or wherever you get your podcasts 👇
Go deeper…

Join 10,000+ designers
Get our weekly breakdowns

"There's no doubt that Dive has made me a better designer"
@ned_ray

Join 10,000+ designers
Get our weekly breakdowns

"There's no doubt that Dive has made me a better designer"
@ned_ray

Join 10,000+ designers
Get our weekly breakdowns

"There's no doubt that Dive has made me a better designer"
@ned_ray



hello@dive.club
Ⓒ Dive 2024